Demystifying the Digital Minds: Machine Learning vs. Artificial Intelligence
Author: Tech Wealth Buzz
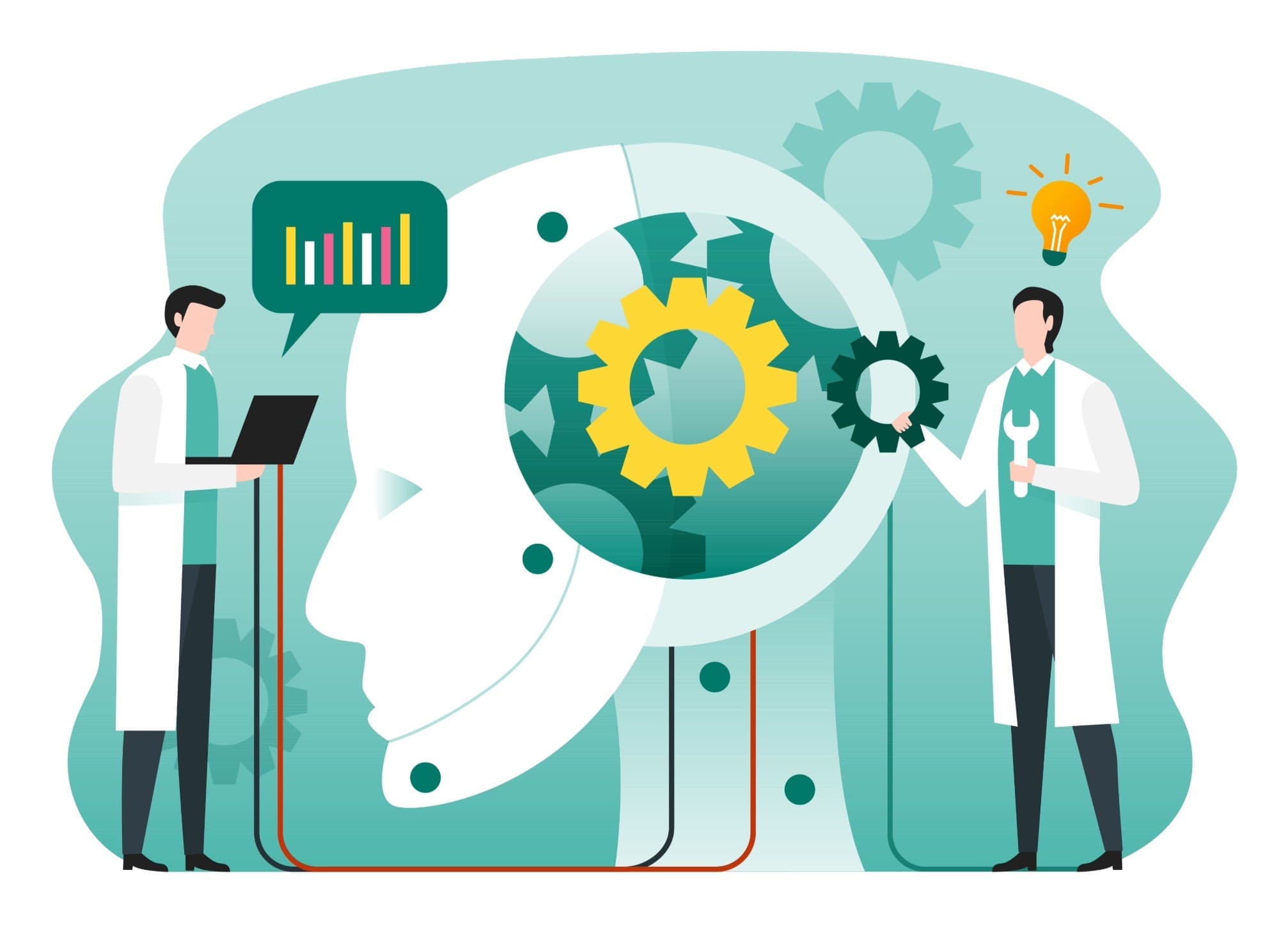
In the realms of technology, two buzzwords often take center stage: Machine Learning (ML) and Artificial Intelligence (AI). While they are closely related, they are not synonymous. In this post, we'll embark on a journey to unravel the distinctions between these two powerful concepts, helping you gain a clearer understanding of their roles in our digital world.
The Essence of Artificial Intelligence (AI):
AI is the overarching concept - a broad field of computer science focused on creating machines or systems capable of performing tasks that typically require human intelligence. AI aims to build machines that can perceive their environment, reason, learn from experience, and make decisions.
Key Attributes of AI:
Generalization:
AI systems possess the ability to generalize knowledge learned from one task and apply it to similar, yet distinct, tasks. In other words, AI has the potential to adapt and solve various problems.
Human-like Intelligence:
The ultimate goal of AI is to mimic human intelligence, allowing machines to understand, interpret, and interact with the world in ways that resemble human cognition.
Complex Decision-Making:
AI systems are capable of complex decision-making, often in real-time, across a wide range of domains, from autonomous vehicles to medical diagnosis.
The Essence of Machine Learning (ML):
Machine Learning, on the other hand, is a subset of AI. It's a method by which AI systems learn from data and improve their performance on a specific task over time without being explicitly programmed. ML relies on algorithms and statistical models to find patterns, make predictions, or optimize decisions.
Key Attributes of Machine Learning:
Data-Driven:
ML systems depend on data for learning. The more data they have, the better they become at making predictions or decisions.
Specific Tasks:
ML is designed to excel in specific tasks, such as image recognition, natural language processing, recommendation systems, and more.
Training and Feedback:
ML models require training, during which they learn from labeled data. They improve their performance through feedback loops and iteration.
Differentiating ML from AI:
Scope:
AI is a broader concept that encompasses any machine or system designed to simulate human-like intelligence, while ML is a subset of AI that focuses on learning from data.
Human-Like vs. Data-Driven:
AI aims to replicate human-like intelligence, whereas ML is more data-driven, focusing on making predictions or decisions based on patterns in data.
General vs. Specific Tasks:
AI has the potential to handle a wide range of tasks and adapt to new ones, while ML specializes in particular tasks and becomes better at them with experience.
Adaptability:
AI can exhibit adaptability across various domains and tasks, while ML models need explicit training for each task they perform.
Conclusion:
Machine Learning and Artificial Intelligence are interconnected but distinct concepts in the world of technology. While AI is the broader goal of creating human-like intelligence in machines, ML is the practical approach that enables machines to learn from data and improve their performance in specific tasks. Understanding these differences is essential for harnessing their power and applying them effectively in various industries and applications. Whether you're navigating the AI landscape or diving into the world of ML, both have transformative potential that continues to shape our digital future. 🤖🧠🌐 #AI #MachineLearning